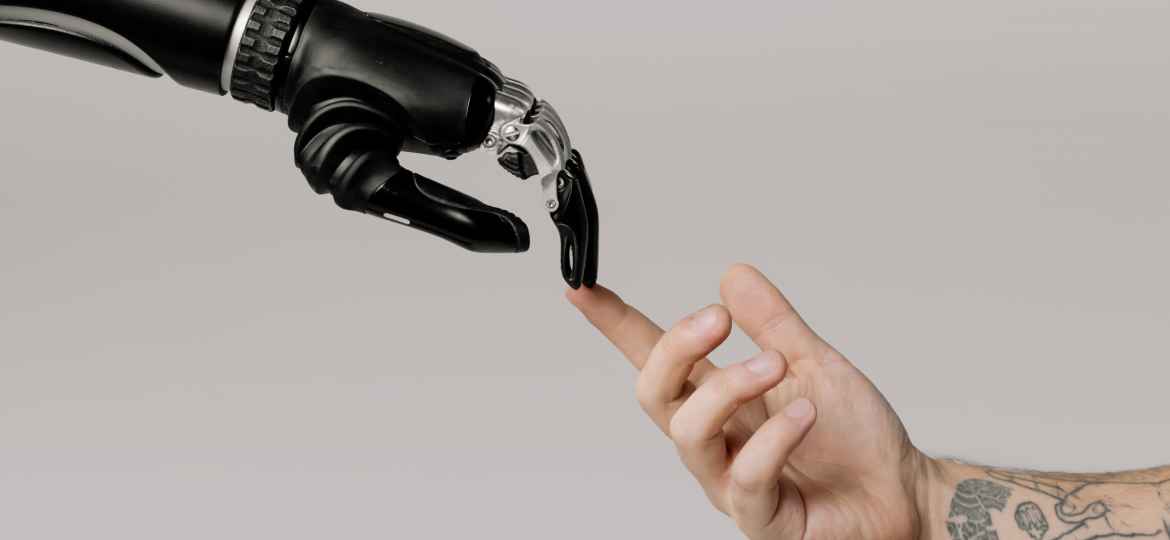
TANGO BLOGPOST
As PhD students at the Technical University Darmstadt, we, Tim Tobiasch and Wolfgang Stammer, are excited to be a part of the EU Horizon research project, “Tango”. Our work focuses on the development and investigation of the development of synergistic AI systems that support human-machine decision making. This blog post aims to highlight our recently published research in this area.
Vision-Language Models: Still Far from True Visual Reasoning
One of our research areas explores how well Vision-Language Models (VLMs) understand and reason about the visual world by combining what they ‘see’ with what they ‘read’. These models, such as the advanced GPT-4, have shown impressive abilities in handling various tasks. But the big question remains: can they truly grasp visual concepts and reason in a way that resembles human understanding?
Figure 1: VLMs struggle to solve BPs out of the box. Although the concepts of vertical and horizontal may seem trivial to a human, common VLMs struggle to generate discriminative rules.
To investigate this, we used Bongard Problems (BPs), a type of classical visual puzzle that requires the detection of patterns and the deduction of abstract rules. Each puzzle contains two groups of images, each governed by a unique rule (see Figure 1). The task is to identify the rule that separates one group from the other. Surprisingly, even apparently simple distinctions, such as recognizing a spiral shape, proved challenging for these advanced models.
Our results show that VLMs, even the best performing ones, struggle with Bongard Problems. For example, GPT-4 could only solve 21 out of 100 puzzles, suggesting that these models have difficulty perceiving and understanding basic visual concepts. These limitations highlight a gap in visual reasoning, and emphasize the importance of combining human insight with machine learning to push the boundaries of AI.
Neural Concept Binder: Towards Unsupervised Concept Learning
Figure 2: Trustworthy learning of concepts for visual reasoning requires that a model provide inspectable and revisable concept representations. In this example, the AI learns from images of multiple objects and creates unambiguous, revisable concepts so that humans can understand and correct its understanding as needed.
One exciting area of research in the Tango project is helping AI learn concepts without needing direct human guidance, a process known as unsupervised concept learning. Imagine an AI system looking at a set of images with various objects—like colorful balls and cubes—and figuring out on its own what those objects are, without any labels or instructions. This kind of learning could lead to AI systems that understand and interact with the world more independently. However, teaching a machine to understand these things without any guidance is no small feat—it needs to form clear and useful ideas about what it “sees” (see Figure 2).
The Neural Concept Binder (NCB) framework addresses this challenge by combining two essential techniques: “soft binding” and “hard binding.” Soft binding helps the AI separate and recognize individual objects in an image, while hard binding organizes these objects into distinct categories, or concepts, that humans can review and adjust as needed. For example, if the AI mistakes a blue ball for a blue cylinder, a human can easily correct it, helping the system become smarter over time.
This approach allows NCB to learn clear, flexible concepts from unlabeled images, creating a system that can adapt to new tasks. Our tests show that NCB performs well even at complex tasks that require reasoning, such as solving visual puzzles, and is nearly as accurate as AI systems that start with labeled data. Best of all, NCB’s design allows humans to review and adjust its understanding, making it a powerful tool that combines independent learning with human guidance.
Our work on Vision-Language Models (VLMs) and the Neural Concept Binder (NCB) within the Tango project reflects our dedication to enhancing collaboration between humans and AI. By building AI systems that can learn, reason, and communicate in a transparent and understandable way, we aim to bridge the gap between machine intelligence and human insight. We believe this approach will enable AI to tackle complex real-world problems more effectively, unlocking its full potential to make a positive impact.
Written by: PhD students at the Technical University Darmstadt, Tim Tobiasch and Wolfgang Stammer